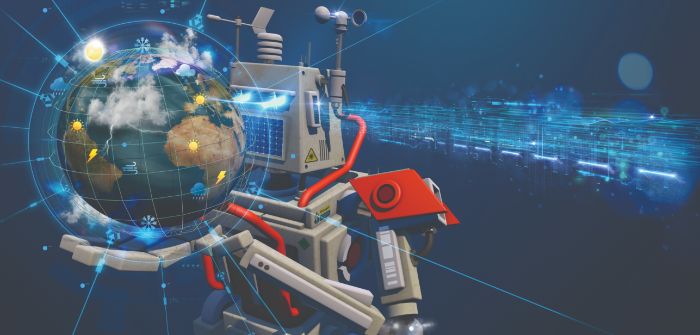
Credit: Sean Rodwell
From Meteorological Technology Int. by Helen Norman
The meteorological sector has been using artificial intelligence (AI) for nearly half a century, according to Dr Peter Neilley, director of weather science and technologies at The Weather Company, an IBM Business.
“AI is not a ‘Johnny-come-lately’ to the atmospheric sciences,” he says.
“It has been ingrained in how we do our business since before the term AI was even invented.”
Neilley recalls a professor called Harry Glahn, who back in the 1970s came up with the model output statistics (MOS) system, which took numerical weather prediction (NWP) outputs and used machine learning (ML) – a subset of AI – to make the data better.
“That technique has been integral to how weather forecasting has been carried out for years,” explains Neilley.
“The foundational concept of taking output from physical NWP models and improving on them is still arguably the number-one use of ML and AI in our sciences today.”
What has changed, however, is the sophistication of the process, Neilley notes: “The statistical and mathematical tools that we have available to work with today, and the computing tech that we use to apply it, have been improved greatly over many generations.”
The Weather Company uses AI in almost everything it does today.
“It’s foundational to optimized weather forecasting and to the translation of that information into the products and services we put out,” explains Neilley.
Its use of AI has enabled The Weather Company to be rated “the overall most accurate provider globally” in 2023 by ForecastWatch, an organization that evaluates the accuracy of weather forecasts.
In fact, The Weather Company has been the world’s most accurate forecaster overall every year since the study began in 2017.
The Weather Company uses AI as a critical tool for meteorologists to make sense of billions of unstructured weather datapoints produced by satellites, ground sensors, radars, models and more.
With advanced ML algorithms and computing power from IBM, meteorologists at The Weather Company can use this data to simulate atmospheric conditions and better forecast the weather, in real time.
“This is basically the MOS process, but on steroids,” Neilley comments.
Emerging uses for AI
The accuracy of forecasts within IBM’s weather products is critical not only for keeping consumers informed, but also for delivering AI-based insights and decision capabilities across industries.
This will be a big area of growth for AI and weather in the future, according to Neilley.
“This approach turns the weather forecast into information that matters to you and your business,” he explains.
“For example, using AI to analyze weather data and create insights on things such as what you should wear, what time you should leave for work, or how successful a specific event will be.
Weather impacts all these things and many more.
I believe we will see explosive growth in this area.
We are just on the cusp of understanding the possibilities of this and there really are billions of possibilities.
AI is going to help us touch many more of those intersections between weather and optimized societal decisions.”
Another emerging use for AI in meteorology is using the technology to replace physical NWP models, such as the ones used by ECMWF or the Global Forecast System (GFS).
“These models use physics to predict what the forecast will be, and then AI is often applied on top to make the forecast better and to create insights,” explains Neilley.
“But now we are seeing an emerging science that could replace those physics-based NWP models with AI-based NWP models.
This would bring AI all the way back to the beginning of the forecast process.”
Neilley notes that The Weather Company is “very excited” by this capability, but stresses that the approach is still “immature” and is currently “not as good as the physical models”.
He adds, “AI-based NWP models are not ready for prime time yet, except maybe in some exceptional use cases such as long-term climate simulations, but the pace at which they have improved over the past few years is remarkable.”
The Weather Company is paying close attention to developments in this area, including developing partnerships with some of the key players in the space, such as Nvidia’s Earth-2 digital twin of Earth’s climate.
The next big thing?
One of the newest entrants in the AI-based NWP model space is GraphCast, which has been developed by Google DeepMind, an AI research lab.
“GraphCast is a weather simulator based on ML and graphical neural networks, using a novel high-resolution multiscale mesh representation.
It forecasts five surface variables and six atmospheric variables, at 0.25° resolution and 37 vertical pressure levels, and can produce a 10-day forecast in less than a minute on a single Tensor Processing Unit (TPU),” explains Matthew Wilson (right), senior research engineer from the Google DeepMind GraphCast team.
GraphCast has been trained on and verified against reanalysis data from ECMWF’s ERA5 data set.
ERA5 is the fifth-generation ECMWF atmospheric reanalysis of the global climate, covering the period from January 1950 right up to the present day.
“We found GraphCast to be more accurate than ECMWF’s deterministic operational forecasting system, HRES, on 90% of the thousands of variables and lead time combinations we evaluated,” says Wilson.
A further comprehensive verification of GraphCast’s forecasts is currently under journal review.
The team is also exploring a range of deployment scenarios.
According to Wilson, GraphCast addresses the challenge of medium-range forecasting at high vertical and horizontal resolution “with greater accuracy than NWP forecasts and with significantly less computational cost”.
He continues, “Its improved accuracy is a result of learning from observations – via reanalysis data – rather than relying on hand-tuned parameterization schemes, together with the strong predictive capacity of a neural network architecture that has been tailored for the geospatial domain.”
The team’s focus in the development of GraphCast has been on deterministic forecasts of medium-range weather, however, it believes the model architecture will generalize well to other geospatial forecasting problems.
“We’re excited to apply the GraphCast architecture to a wider range of problem settings.
Watch this space,” he adds.
Cost implications
As Wilson mentioned, a key benefit of the GraphCast solution is reduced cost compared to traditional NWP forecast models.
Nvidia, which has a strong presence in the use of AI for meteorology, also believes that cost is a big benefit of AI weather prediction.
Dion Harris, lead product manager of accelerated computing at Nvidia, explains, “Traditional numerical simulations on supercomputers can be computationally intensive and expensive, requiring significant resources and infrastructure.
In contrast, AI techniques such as ML can leverage existing computational resources more efficiently, reducing costs.
AI can provide accurate and fast predictions at a lower computational cost, making it an attractive alternative for certain applications in meteorology.”
Nvidia’s Fourier ForeCasting Neural Network (FourCastNet) is a physics-ML model that emulates the dynamics of global weather patterns and predicts extremes with “unprecedented” speed and accuracy.
FourCastNet is GPU-accelerated, powered by the Fourier Neural Operator, and trained on 10TB of Earth system data.
“Each seven-day forecast requires only a fraction of a second on a single Nvidia GPU to generate potential impacts based on thousands of scenarios.
That’s five orders of magnitude faster than numerical weather predictions,” explains Harris.
He also notes how the model can compute a 100-member ensemble forecast using vastly fewer nodes, at a speed that is between 45,000 times faster (at 18km resolution) and 145,000 times faster (at 30km resolution) on a node-to-node comparison.
By the same estimates, FourCastNet has an energy consumption that is between 12,000 (18km) and 24,000 (30km) times lower than that of the Integrated Forecasting System model.
“However, it’s important to note that the development and training of AI models also require significant computational resources, expertise and data, which can incur costs,” Harris continues.
“The affordability of AI versus supercomputers depends on the specific use, the scale of the problem, the available resources and the required accuracy and precision.”
“The affordability of AI versus supercomputers depends on the specific use, the scale of the problem, the available resources and the required accuracy and precision.”
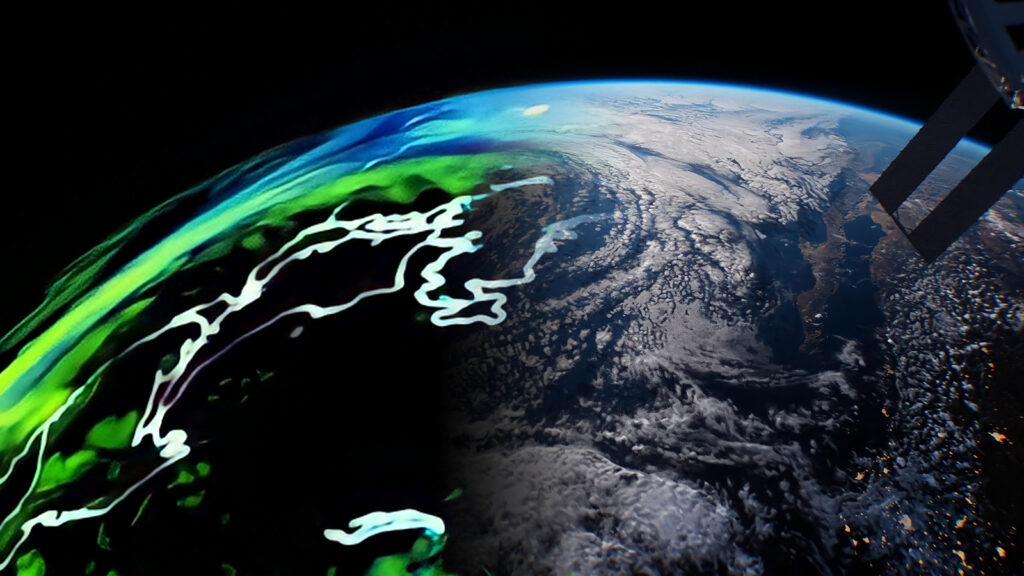
Pic: Nvidia
Digital twins
Nvidia is currently eight months into the two-year NOAA Earth Observations Digital Twin (EODT) project, which seeks to build an AI-driven digital twin for Earth observations.
The prototype system will ingest, analyze and display terabytes of satellite and ground-based observation data from five Earth system domains: atmosphere (temperature and moisture profiles), ocean (sea surface temperature), cryosphere (sea ice concentration), land and hydrology (fire products) and space weather (solar wind bulk plasma).
Nvidia is working closely with Lockheed Martin Space on the EODT project, which will essentially showcase how NOAA could use AI/ML and digital twin technology to optimize the vast amount of data the agency receives from multiple ground and space data sources.
It will provide an extremely accurate, high-resolution depiction of current global weather conditions.
As part of the project, Lockheed Martin’s OpenRosetta3D platform uses AI/ML to ingest, format and fuse observations from multiple sources into a gridded data product to detect anomalies.
The platform also converts this data into a USD (universal scene description) format.
Nvidia’s Omniverse Nucleus platform will connect multiple applications to a real-time 3D environment and serve as a data store and distribution channel, enabling data sharing across multiple tools and between researchers.
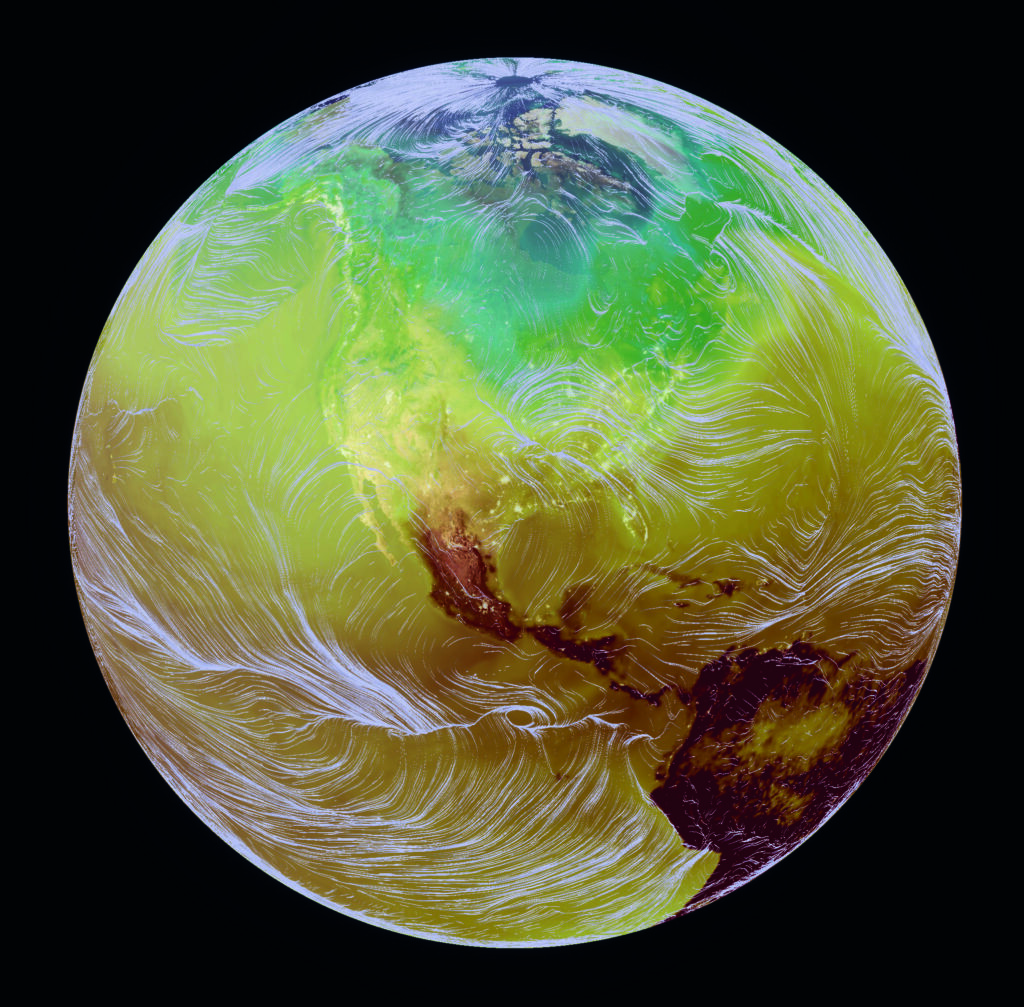
Speaking about progress so far, Lynn Montgomery, Lockheed Martin science researcher for EODT, says, “In December 2022, the project began ingesting data from the Geostationary Operational Environmental Satellite-R (GOES-R) series, and the Suomi National Polar-orbiting Partnership (NPP) Satellite.
We have been able to format, grid and tile the data as well as pass it into an Omniverse Nucleus instance where it can be fed into our front-end user interface and visualized.”
As of May 2023, the EODT team has been working on developing custom AI/ML algorithms for data fusion and anomaly detection, as well as working to make sure it has a scalable and stable framework.
By September 2023, one year into the two-year contract, Lockheed Martin and Nvidia expect to fully integrate and demonstrate one of the variable data pipelines – sea surface temperature.
“This alone is an incredible amount of data,” Montgomery continues.
“The EODT team is well on its way to demonstrating how AI/ML can seriously help humans deal with and use the increased amount of data at our fingertips.
For NOAA, this prototype will show a more efficient and centralized approach for monitoring current global weather conditions.”
Besides the ability to fuse data from multiple diverse sources, AI/ML can play an important role in detecting anomalies.
“That’s a good thing,” Montgomery notes.
“Anomalies represent instances in data where something is unusual or unexpected.
In NOAA’s case it might be a spike in ocean temperature on a sensor, revealing a calibration error or an actual physical anomaly.
The AI/ML can sort through all the data and call attention to something like that, which would warrant further human examination."
Links :
- SiliconRepublic : How AI is being used to improve weather forecasts
- China Daily : China uses AI for enhanced precision in forecasting extreme weather
- Scientific Computing World : AI weather forecasting model now publicly available
- CNN video : How A.I. is changing weather forecasting
No comments:
Post a Comment