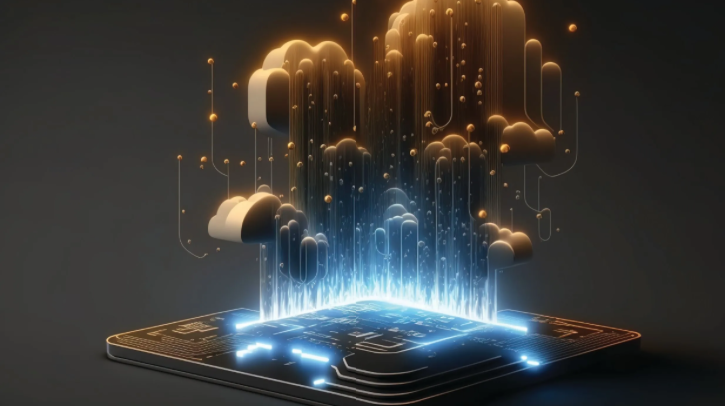
From Meteorological Technology Int by Jack Roper
Generative artificial intelligence could transform the meteorological sector if issues of data availability and accuracy, model trust and lack of transparency are overcome.
Like virtually every sector, meteorology may soon find itself transformed by generative artificial intelligence (GAI), a technology that UN secretary-general António Guterres believes has enormous potential for good and evil at scale.
Even before the advent of GAI, AI models have shown predictive capability competitive with numerical weather prediction (NWP).
“Physics-based forecast models take observations of the atmosphere’s current state then use our knowledge of physics to move them forward in time,” explains Kyle Hilburn, a research scientist at Colorado State University’s Cooperative Institute for Research in the Atmosphere (CIRA).
“AI models learn from training data then use that learning, rather than physics, to move observations forward.”
Efforts to use machine learning to replace problematic parameterizations of subscale processes such as turbulence and cloud physics in NWP models date back to the 1990s.
But only in 2018 did Peter Dueben and Peter Bauer, who both work on Earth system modeling at the ECMWF, first posit the feasibility of entirely replacing NWP models with AI.
“From 2022 onward, big tech companies made breakthroughs in AI weather prediction and began sharing their findings,” says Dr Simon Driscoll, who researches AI weather and climate modeling at the University of Reading.
“The last two years have seen an explosion in AI models producing fast and accurate forecasts that rival and sometimes outperform NWP models.”
This wave of NWP-competitive AI models includes Google’s GraphCast, which uses machine learning in place of numerical physics to improve medium-range forecasts, and Nvidia’s FourCastNet model based on Fourier neural operators, a machine learning technique tailored to physical systems governed by partial differential equations.
“FourCastNet has proved as skilful as state-of-the-art NWP, but 10,000 to 100,000 times faster and more energy efficient,” says Nvidia’s director of accelerated computing products, Dion Harris.
“It enables generation of massive ensembles to more accurately characterize the probabilities of low-likelihood, high-impact events.
Its latest version uses spherical harmonics, making possible prediction over decades or even centuries.”
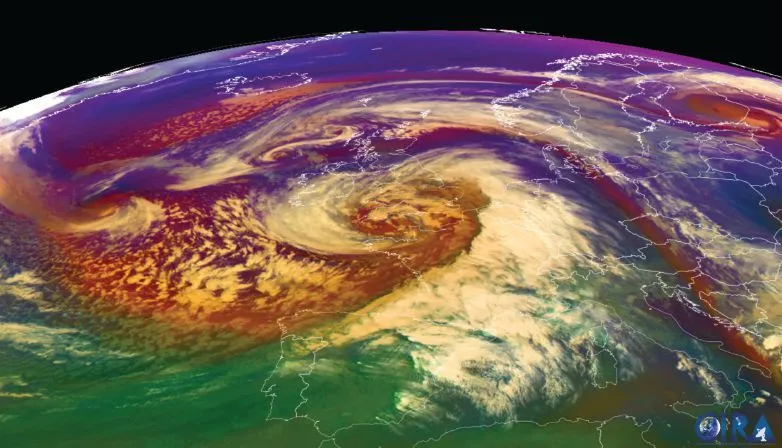
Testing, testing
University of Reading scientists tested the ability of AI models including GraphCast and FourCastNet to predict the development of Storm Ciarán, which brought death and disruption to northwestern Europe in late 2023.
The models were initialized with ECMWF data from an early stage in Ciarán’s development and tasked with replicating its evolution.
“The models were good at tracking the storm’s position and capturing variables such as mean sea-level pressures,” comments Driscoll.
“But they were less good at things we truly care about, such as peak gust speeds, which relate to damage.
It suggests that naïve use of these models could lead us to issue the wrong level of weather warning, resulting in insufficient precautions and increased loss of life,” he adds.
“Predictive AI outputs the most likely or average outcome over a range of possibilities, whereas GAI outputs one possible outcome”
Kyle Hilburn, Cooperative Institute for Research in the Atmosphere (CIRA), Colorado State University
Why AI models may under-represent extreme events is not definitively understood, but one factor may be the under-representation of such events in training data.
Classification algorithms are typically trained on data representing (for instance) cats and dogs in equal proportions, but storms like Ciarán are statistical outliers with fewer examples to learn from.
Another issue may be the metrics used to optimize models.
“Computer scientists optimize models using metrics such as mean-squared error, but a loss function based on the mean won’t be suited to capturing extremes,” comments CIRA’s Hilburn.
“We need to optimize for things meteorologists care about – and non-traditional, object-based metrics could provide better information about extreme events like storms and hurricanes.”
Emerging Gen AI models
Although the atmosphere is a continuous fluid, storms can be considered discrete objects with recognizable perimeters that can be tracked over time.
Optimizing models to track such objects, rather than mean accuracy, could improve their sensitivity to extreme events.
A new generation of models based on generative AI may equally solve the problem.
“GAI learns to generate new content from given inputs,” Nvidia’s Harris explains.
“It learns patterns and structures of entire distributions of data and generates new instances.
It can generate synthetic satellite images that are statistically indistinguishable from the true images it trained on,” he adds.
GAI is the technology behind large language models (LLMs) such as ChatGPT, and can produce text, music or pictures that resemble human outputs in response to prompts.
Meteorologists distinguish generative AI from the predictive AI behind GraphCast and FourCastNet.
“Predictive AI outputs the most likely or average outcome over a range of possibilities, whereas GAI outputs one possible outcome,” explains Hilburn.
“The average may not be meaningful in relation to extreme events, or scenarios with two divergent possibilities.
GAI diffusion models, trained to remove noise from images and learn about underlying structures, seem to provide sharper, more realistic features than other types of AI.”
Predictive models respond to uncertainty with less specific predictions; GAI models choose one possibility to assemble into an outcome.
Repeating this process many times could create large ensembles to represent the probability of extreme events developing from a given atmospheric state.
This is the aim of Google’s Scalable Ensemble Envelope Diffusion Sampler (SEEDS) model.
“Current best practice in ensemble weather prediction is to run a weather model 30 to 50 times on a supercomputer with slightly different initial conditions,” explains Google research scientist Rob Carver.
“If it rains in 50% of those forecasts, the probability of rain is 50%.
By generating hundreds or thousands of forecasts in record time, GAI can model probability more accurately than the best traditional methods.”
By multiplying ensemble members showing the possible range of events in the next 3 to 10 days, SEEDS may improve the statistical accuracy of forecasts, enabling the likelihood of events like Storm Ciarán to be better assessed.
“We base the chance of a weather event on how many of our forecasts show it will happen, so more forecasts means greater accuracy.
Running traditional models takes hours or days.
SEEDS cuts that time by 90% and produces as many forecasts with 90% less computing power,” Carver adds.
The butterfly effect
Computational cost is a limiting factor for physics-based ensembles, as is the slowness of NWP models when end users need to know conditions in the next two hours.
Although expensive to train, GAI models cost little to run once trained and may produce forecasts in seconds rather than hours.
“Running traditional models takes hours or days.
SEEDS cuts that time by 90%.”
Rob Carver, Google
“GAI ensemble forecasts may be the next domain for tech companies,” says Driscoll.
“Because the atmosphere is chaotic, small perturbations in initial conditions lead to wildly divergent predictions after a few days.
GAI models can produce forecasts with an enormous number of initial conditions and sample initial uncertainty extremely quickly,” he adds.
Ensembles address the so-called butterfly effect, whereby small initial errors grow into large errors and cause the accuracy of weather models to deteriorate over time.
But questions remain as to whether GAI can truly decipher the inherent uncertainty of weather.
University of Reading scientists tested the ability of AI models including GraphCast and FourCastNet to predict the development of Storm Ciarán, which brought death and disruption to northwestern Europe in late 2023.
The models were initialized with ECMWF data from an early stage in Ciarán’s development and tasked with replicating its evolution.
“The models were good at tracking the storm’s position and capturing variables such as mean sea-level pressures,” comments Driscoll.
“But they were less good at things we truly care about, such as peak gust speeds, which relate to damage.
It suggests that naïve use of these models could lead us to issue the wrong level of weather warning, resulting in insufficient precautions and increased loss of life,” he adds.
“Predictive AI outputs the most likely or average outcome over a range of possibilities, whereas GAI outputs one possible outcome”
Kyle Hilburn, Cooperative Institute for Research in the Atmosphere (CIRA), Colorado State University
Why AI models may under-represent extreme events is not definitively understood, but one factor may be the under-representation of such events in training data.
Classification algorithms are typically trained on data representing (for instance) cats and dogs in equal proportions, but storms like Ciarán are statistical outliers with fewer examples to learn from.
Another issue may be the metrics used to optimize models.
“Computer scientists optimize models using metrics such as mean-squared error, but a loss function based on the mean won’t be suited to capturing extremes,” comments CIRA’s Hilburn.
“We need to optimize for things meteorologists care about – and non-traditional, object-based metrics could provide better information about extreme events like storms and hurricanes.”
Emerging Gen AI models
Although the atmosphere is a continuous fluid, storms can be considered discrete objects with recognizable perimeters that can be tracked over time.
Optimizing models to track such objects, rather than mean accuracy, could improve their sensitivity to extreme events.
A new generation of models based on generative AI may equally solve the problem.
“GAI learns to generate new content from given inputs,” Nvidia’s Harris explains.
“It learns patterns and structures of entire distributions of data and generates new instances.
It can generate synthetic satellite images that are statistically indistinguishable from the true images it trained on,” he adds.
GAI is the technology behind large language models (LLMs) such as ChatGPT, and can produce text, music or pictures that resemble human outputs in response to prompts.
Meteorologists distinguish generative AI from the predictive AI behind GraphCast and FourCastNet.
“Predictive AI outputs the most likely or average outcome over a range of possibilities, whereas GAI outputs one possible outcome,” explains Hilburn.
“The average may not be meaningful in relation to extreme events, or scenarios with two divergent possibilities.
GAI diffusion models, trained to remove noise from images and learn about underlying structures, seem to provide sharper, more realistic features than other types of AI.”
Predictive models respond to uncertainty with less specific predictions; GAI models choose one possibility to assemble into an outcome.
Repeating this process many times could create large ensembles to represent the probability of extreme events developing from a given atmospheric state.
This is the aim of Google’s Scalable Ensemble Envelope Diffusion Sampler (SEEDS) model.
“Current best practice in ensemble weather prediction is to run a weather model 30 to 50 times on a supercomputer with slightly different initial conditions,” explains Google research scientist Rob Carver.
“If it rains in 50% of those forecasts, the probability of rain is 50%.
By generating hundreds or thousands of forecasts in record time, GAI can model probability more accurately than the best traditional methods.”
By multiplying ensemble members showing the possible range of events in the next 3 to 10 days, SEEDS may improve the statistical accuracy of forecasts, enabling the likelihood of events like Storm Ciarán to be better assessed.
“We base the chance of a weather event on how many of our forecasts show it will happen, so more forecasts means greater accuracy.
Running traditional models takes hours or days.
SEEDS cuts that time by 90% and produces as many forecasts with 90% less computing power,” Carver adds.
The butterfly effect
Computational cost is a limiting factor for physics-based ensembles, as is the slowness of NWP models when end users need to know conditions in the next two hours.
Although expensive to train, GAI models cost little to run once trained and may produce forecasts in seconds rather than hours.
“Running traditional models takes hours or days.
SEEDS cuts that time by 90%.”
Rob Carver, Google
“GAI ensemble forecasts may be the next domain for tech companies,” says Driscoll.
“Because the atmosphere is chaotic, small perturbations in initial conditions lead to wildly divergent predictions after a few days.
GAI models can produce forecasts with an enormous number of initial conditions and sample initial uncertainty extremely quickly,” he adds.
Ensembles address the so-called butterfly effect, whereby small initial errors grow into large errors and cause the accuracy of weather models to deteriorate over time.
But questions remain as to whether GAI can truly decipher the inherent uncertainty of weather.
“It remains unclear whether GAI models really represent the butterfly effect,” says CIRA’s Hilburn.
“Where physics-based models show that error growth over time, GAI models seem to stick to the same solution as they’re integrated forward.
We can run huge GAI ensembles but if those models aren’t diverging, they may not predict the full range of possibilities.”
Since GAI and physics-based models exhibit different error characteristics, Hilburn foresees value in ensembles combining both model types.
Google’s SEEDS remains an internal research project today and further research must determine whether GAI can be relied on for actionable weather information.
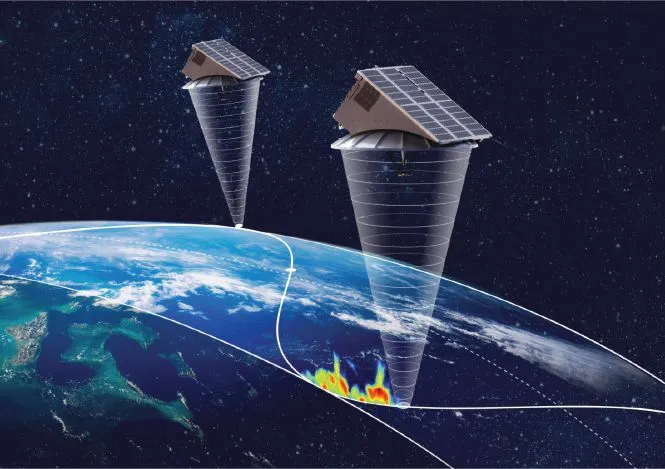
Building trust
While meteorologists use their knowledge of physics to ascertain whether physics-based forecasts make sense, establishing trust in the inscrutable learning behind GAI predictions presents a different challenge.
“Explainable AI techniques can help users understand and trust AI outputs,” says Driscoll.
“Symbolic regression uses machine learning to search for mathematical expressions that make model outputs human-readable.
That reduces the black-box problem but is hard to apply to weather forecasts, given their complexity.”
“Explainable AI techniques can help users understand and trust AI output.”
Dr Simon Driscoll, University of Reading
GAI can also ‘hallucinate’ erroneous solutions – for example, when satellite imagery lacks sharp patterns or gradients pointing to any definite outcome.
Rather than admit to uncertainty, Hilburn says current models venture a potentially incorrect answer, providing an unsound basis for safety-critical decisions.
“Diagnostic tools could be used to filter out unphysical, grossly inaccurate or clearly impossible hallucinated predictions,” Harris suggests.
“Physics-derived ‘guardrails’ and techniques such as domain alignment could refine GAI to provide information better-suited to exact applications.”
Generally speaking, the predictive power of GAI models increases in proportion to the quantity and richness of training data.
Harris sees short supply of such data as the greatest single limitation on GAI forecasting capability today.
“High-quality, ultra-high-resolution data is not easily available in vast amounts,” he says.
“This data challenge requires us to fuse multiple, complementary sources of data and develop novel means of data augmentation, perhaps using synthetic data from physical models.”
Collaboration is essential
GAI presents well-publicized potential to replace human jobs in many sectors and may soon be writing articles such as this.
But rather than rendering meteorological observations obsolete, its thirst for training data will drive an increased demand for data.
Although it may transform how forecasts are made, realizing its potential demands cooperation between developers and meteorologists.
“For AI models to help humanity, we need transparency and reproducibility,” comments Hilburn.
“But private companies may not have an incentive to make models publicly available.
So far, evaluation has primarily been by AI developers themselves.
What developers think is important is not necessarily the same as what meteorologists think is important,” he adds.
GAI presents well-publicized potential to replace human jobs in many sectors and may soon be writing articles such as this.
But rather than rendering meteorological observations obsolete, its thirst for training data will drive an increased demand for data.
Although it may transform how forecasts are made, realizing its potential demands cooperation between developers and meteorologists.
“For AI models to help humanity, we need transparency and reproducibility,” comments Hilburn.
“But private companies may not have an incentive to make models publicly available.
So far, evaluation has primarily been by AI developers themselves.
What developers think is important is not necessarily the same as what meteorologists think is important,” he adds.
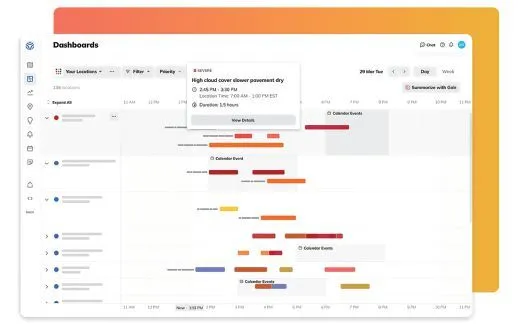
Tomorrow.io’s Resilience Platform
In the US, the AI Institute for Research on Trustworthy AI in Weather, Climate, and Coastal Oceanography (AI2ES) exists to bridge this gap between computer and climate science.
Meanwhile, the ECMWF demonstrates leadership in developing AI-based models alongside established, physics-based capabilities.
“The pace of development has left many institutes out of the loop,” says Driscoll.
“But the ECMWF has responded very quickly and already runs an AI model alongside operational forecasts, demonstrating that forecasting centers can keep up with the tech giants.”
GAI’s meteorological applications could include boosting ensembles, qualifying uncertainty and downscaling image resolution.
It also promises to improve resilience and decision making by distilling complex forecast information into concise and actionable summaries.
This is the aim of Gale, a GAI assistant available to customers of weather intelligence provider Tomorrow.io (see Developing an all-seeing radar constellation to advance Gen AI, page 12).
“Gale summarizes and condenses information in a readable, shareable way,” says Tomorrow.iogrowth and strategy VP Cole Swain.
“Where once we did data visualizations, chatting to Gale gets the answer you need right away.
Because it combines forecast information with our foundational understanding of an enterprise’s problems and protocols, it can say, ‘Hey, Jimmy – reschedule your rooftop solar installation on Tuesday because there’s gonna be heavy rain.’”
Valuable market insights
Rather than developing its own large language model, Tomorrow.io leverages third-party LLMs to enable Gale’s functionality.
Gale uses only one LLM per customer session, but switches between LLMs depending on the required workflow.
“Gale is model-agnostic,” says Swain.
“Some LLMs are better at logic, creating human-readable text or condensing vast amounts of information into something concise.
We have different arrows in our quiver – and our technical stack uses pre-programmed logic to put the right technology in our bow at the right time.”
Tomorrow.io’s customers use the company’s platform to make operational and safety-critical decisions, but their onboarding promotes an understanding that weather is hard to predict.
Gale is accompanied by a disclaimer stating that Tomorrow.io is not responsible for its outputs, and it is discouraged from offering potential hallucinations.
“Our core principle is never to allow the LLM to come up with information itself,” says Swain.
“We use it solely to translate, compress and organize technical, programmatic information into something legible.
Of course, we also take precautionary measures internal to our databases, to validate quality control and assurance.”
Many Tomorrow.io users have embraced Gale.
Following a productive discovery phase, it is in use with selected customers, though the company is mindful to avoid displacing what works by forcing adoption on everyone.
Already, Gale is providing valuable market insights.
“Understanding what customers want is the holy grail of product management,” says Swain.
“Because customers ask Gale questions, we see exactly what they want from our platform.
That understanding of value both accelerates and removes risk from our strategic decisions.”
“Understanding what customers want is the holy grail of product management.”
Cole Swain, Tomorrow.io
The pace of AI revolution is unfamiliar to conventional academic research, with breakthroughs expected to continue at breakneck speed as advances like the Nvidia Grace Hopper AI chip enable bigger models capable of faster data exchange.
“We expect AI to become integrated across the pipeline of operational meteorology, in data fusion, data assimilation, forecasting, post-processing and meaningful, easy-to-understand textual and visual descriptions of weather,” Nvidia’s Harris adds.
“Its potential to enhance meteorological outputs in previously unimaginable ways will be best realized collaboratively, with expert meteorologists in the loop,” he concludes.
Developing an all-seeing radar constellation to advance Gen AI
Tomorrow.io works to build weather resiliency programs with a customer base of hundreds of enterprises and governments worldwide.
Energy companies and airports upload their assets, field staff and schedules onto Tomorrow.io’s cloud-native Software as a Service platform and build protocols to navigate different weather events.
“We predict the intersection of weather with your operations,” says Tomorrow.io’s Cole Swain.
“Instead of just a forecast, we communicate who needs to do what to navigate the problem.”
The company was conceived when its founders devised novel means to infer weather observations from the attenuation of microwave signals and began providing forecasts by aggregating data from government models and sensors worldwide.
“We eventually realized the only way to truly disrupt the industry was to go into space,” Swain recalls.
“Where 90% of the world has no radar coverage today, our own constellation of satellites will provide full global coverage.”
Combined with models, this all-seeing radar constellation will improve the predictions Tomorrow.ioprovides to customers while creating a global dataset valuable in advancing GAI applications in weather and climate.
“Everything in AI is about the inputs,” explains Swain.
“What good is a forecast model if you don’t know the initial conditions? We need observations to train, calibrate and bias-correct AI over time.
That’s where our satellites, providing full atmospheric profiling of every location on Earth at 60-minute intervals, become important to the meteorological industry,” he adds.
The Gale interactive GAI assistant already enables Tomorrow.io users to derive succinct and shareable insights from complex weather reports (see main article).
“Historically, weather is an oratory field,” says Swain.
“People are used to watching the weatherman or reading text-based forecasts.
Now, GAI can summarize how that forecast impacts a user’s operations and they can ask questions about it.
“Across networks with hundreds of locations and thousands of employees, weather events happen every single day,” he continues.
“GAI expedites the ability to respond, telling you where on the network to focus attention and what you need to do next.”
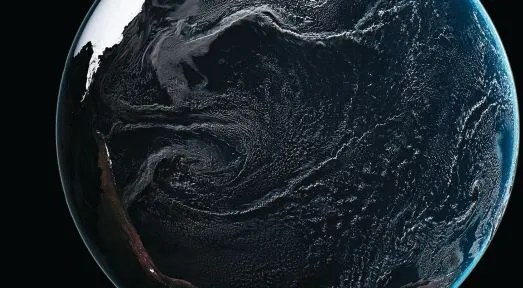
Digital visualization of Nvidia’s Earth-2 software, which will simulate the planet’s climate
Improving the resolution and precision of forecasts
Generative AI can synthesize ultra-high-resolution images by learning the mapping between low-and higher-resolution images – a process called super-resolution in computer science and downscaling in meteorology.
CorrDiff is an Nvidia model that downscales coarse weather data.
“CorrDiff combines a correction model (Corr) and a diffusion model (Diff) to bias-correct and downscale a low-resolution forecast,” explains Nvidia’s Dion Harris.
“It draws on the well-established technique of Reynolds decomposition in fluid dynamics.”
While CorrDiff’s chief purpose is improving the precision and fidelity of forecasts, it also lends itself to infilling or inpainting, data assimilation and synthetic data generation.
Nvidia has proved the concept over a region of Taiwan and the surrounding ocean.
“By training on radar-assimilated Weather Research and Forecasting Model (WRF) data and ECMWF reanalyses, we achieved a 12.5 times increase from 25m to 2km resolution,” Harris reveals.
“CorrDiff generates samples at 2km resolution 1,000 times faster and 3,000 times more energy-efficiently than the WRF numerical weather prediction (NWP) model.
It can also synthesize fields of interest not present in the model inputs, such as radar reflectivity, opening the door to applications like impact assessment.”
Nvidia’s Earth-2 program aims to build the technologies and platforms needed to create digital twins of Earth for weather and climate applications.
Its ultimate goals are twofold: to advance understanding of climate change impacts on hyper-local scales and provide the reliable, actionable information needed to address them.
“Ever-higher-resolution weather and climate data is critically needed in planning for the disastrous consequences of climate change,” adds Harris.
“But simulating our planet at scales of tens of meters with NWP models is computationally very intensive.
By providing 12.5x resolution enhancements 3,000 times more efficiently, CorrDiff represents a major step toward the goals of Earth-2.”
Links :
- GeoGarage blog : Are weather forecasts better with artificial intelligence?
No comments:
Post a Comment