
From Google blog by Sims Witherspoon & Will Fadrhonc
Carbon-free technologies like renewable energy help combat climate change, but many of them have not reached their full potential.
Consider wind power: over the past decade, wind farms have become an important source of carbon-free electricity as the cost of turbines has plummeted and adoption has surged.
However, the variable nature of wind itself makes it an unpredictable energy source—less useful than one that can reliably deliver power at a set time.
In search of a solution to this problem, last year, DeepMind and Google started applying machine learning algorithms to 700 megawatts of wind power capacity in the central United States.
In search of a solution to this problem, last year, DeepMind and Google started applying machine learning algorithms to 700 megawatts of wind power capacity in the central United States.
These wind farms—part of Google’s global fleet of renewable energy projects—collectively generate as much electricity as is needed by a medium-sized city.
This is important, because energy sources that can be scheduled (i.e. can deliver a set amount of electricity at a set time) are often more valuable to the grid.
Although we continue to refine our algorithm, our use of machine learning across our wind farms has produced positive results.
Although we continue to refine our algorithm, our use of machine learning across our wind farms has produced positive results.
To date, machine learning has boosted the value of our wind energy by roughly 20 percent, compared to the baseline scenario of no time-based commitments to the grid.
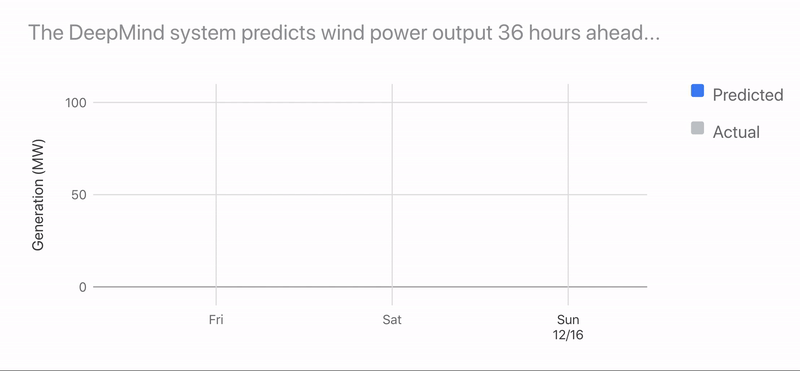
We can’t eliminate the variability of the wind, but our early results suggest that we can use machine learning to make wind power sufficiently more predictable and valuable.
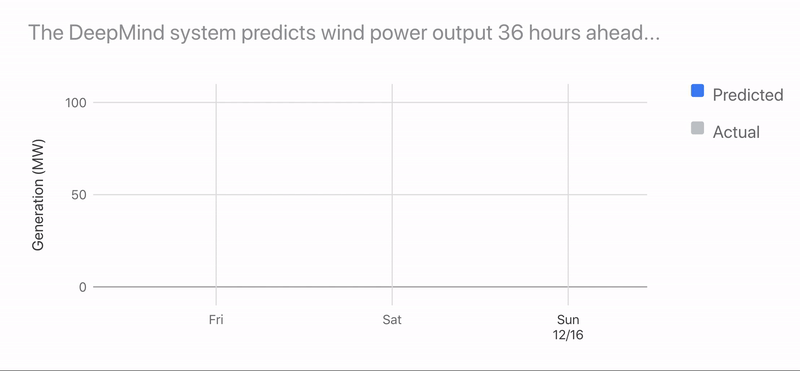
We can’t eliminate the variability of the wind, but our early results suggest that we can use machine learning to make wind power sufficiently more predictable and valuable.
This approach also helps bring greater data rigor to wind farm operations, as machine learning can help wind farm operators make smarter, faster and more data-driven assessments of how their power output can meet electricity demand.

Results from DeepMind application of machine learning to Google’s wind power
Our hope is that this kind of machine learning approach can strengthen the business case for wind power and drive further adoption of carbon-free energy on electric grids worldwide.
Researchers and practitioners across the energy industry are developing novel ideas for how society can make the most of variable power sources like solar and wind.
We’re eager to join them in exploring general availability of these cloud-based machine learning strategies.
Google recently achieved 100 percent renewable energy purchasing and is now striving to source carbon-free energy on a 24x7 basis.
Google recently achieved 100 percent renewable energy purchasing and is now striving to source carbon-free energy on a 24x7 basis.
The partnership with DeepMind to make wind power more predictable and valuable is a concrete step toward that aspiration.
While much remains to be done, this step is a meaningful one—for Google, and more importantly, for the environment.
No comments:
Post a Comment